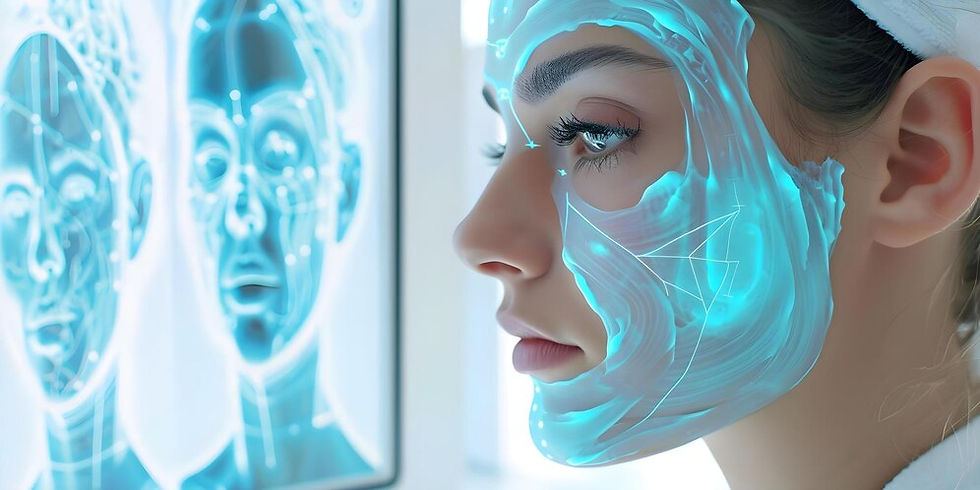
Understanding human emotions and nonverbal cues has always been a crucial aspect of behavioral science. Researchers rely on various tools to decode facial expressions and assess emotional responses. One of the most effective and scientifically backed methods is the Facial Action Coding System. This system offers a standardized approach to analyzing facial movements, making it invaluable for studies in psychology, artificial intelligence, and neuroscience.
What Is the Facial Action Coding System?
The Facial Action Coding System (FACS) is a comprehensive method used to categorize facial movements based on muscle activity. Developed by psychologists, this system breaks down facial expressions into small, measurable units called action units (AUs). These AUs correspond to specific muscle movements that indicate emotions such as joy, sadness, anger, and surprise.
Unlike traditional emotion recognition methods, which often rely on subjective interpretation, FACS provides an objective and scientifically validated framework. This accuracy makes it an essential tool in behavioral science research, particularly in fields like Microexpression Analysis, where detecting fleeting facial expressions is critical.
The Role of FACS in Behavioral Science
1. Enhancing Accuracy in Emotion Research
One of the primary advantages of the Facial Action Coding System is its ability to provide precise and consistent data. Since human emotions are complex, relying on verbal communication alone can lead to inaccuracies. FACS ensures that researchers can capture even the most subtle facial movements, improving the reliability of emotion studies.
This system plays a vital role in Behavioral Response Measurement, helping scientists assess how individuals react to various stimuli. Whether in clinical psychology, marketing, or human-computer interaction, accurate behavioral data is essential for meaningful research outcomes.
2. Supporting AI-Based Emotion Detection
The rise of artificial intelligence has led to the development of AI-Based Emotion Detection systems that rely on facial recognition technology. These AI-driven tools often use datasets trained on FACS principles, ensuring that their analysis of facial expressions is both precise and unbiased.
By integrating FACS into AI models, researchers can improve the accuracy of emotion detection systems, making them more effective in applications such as mental health monitoring, customer experience analysis, and security surveillance. The combination of Microexpression Analysis and AI further enhances the ability to detect hidden emotions that might otherwise go unnoticed.
3. Standardizing Behavioral Response Measurement
For any research method to be widely accepted, it must be standardized and replicable. The Facial Action Coding System provides a universal language for studying facial expressions, making it easier for researchers across different disciplines to compare results.
In fields like neuroscience and psychology, where Behavioral Response Measurement is crucial, FACS ensures consistency in data collection. This standardization reduces biases, increases research credibility, and allows for more accurate cross-cultural studies.
Why FACS Should Be a Standard in Research
Despite its proven benefits, not all behavioral science studies utilize the Facial Action Coding System. Some researchers still rely on less precise methods, which can lead to inconsistent findings. Establishing FACS as a standard would bring several key advantages:
Greater reliability: Objective measurement reduces subjectivity in emotion research.
Improved AI training: Better datasets lead to more accurate AI-Based Emotion Detection.
Enhanced cross-disciplinary use: From psychology to marketing, FACS benefits multiple fields.
As research into human emotions continues to evolve, adopting the Facial Action Coding System as a standard practice will lead to more accurate and insightful discoveries. Whether through Microexpression Analysis or Behavioral Response Measurement, FACS provides the foundation for a deeper understanding of human behavior.
Read more related blogs:
Comments